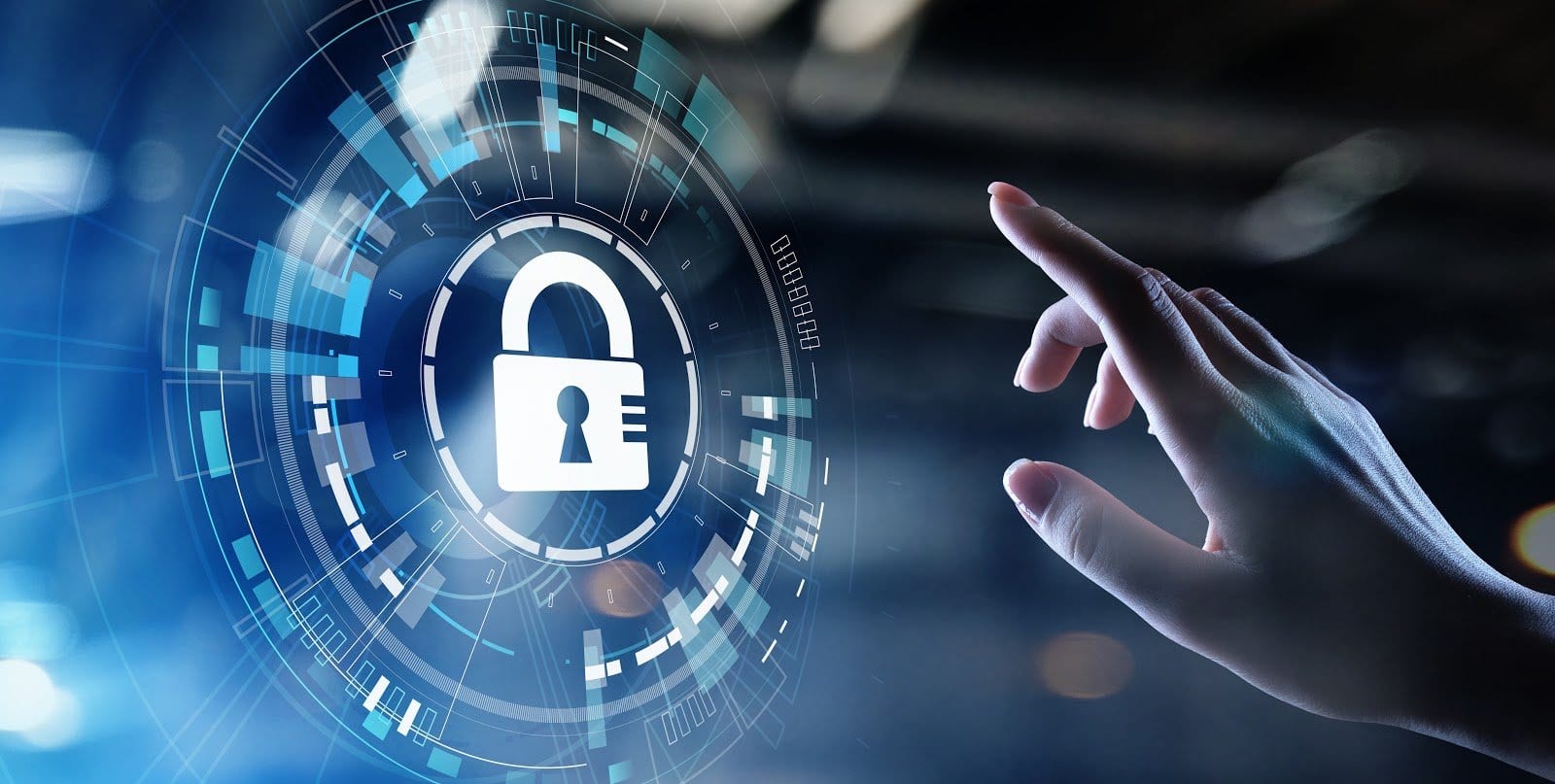
Industry Insights
Predictive Modeling: Protecting Companies Against Digital Fraud
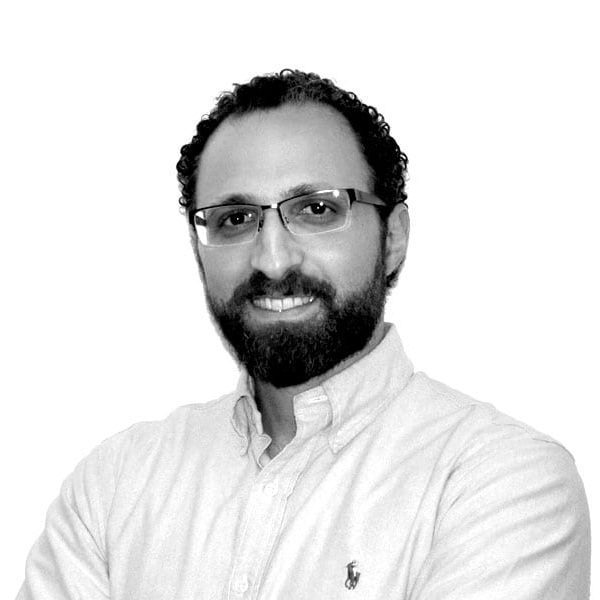
Wassim Chammaa
A seasoned Business Intelligence and Data Warehouse professional with over 15yrs of experience delivering end to end solution in Data Architecture, Analytics Statistical Analysis, and Data Mining and from Reports to Interactive Analysis or Executive dashboards.
December 11th, 2019
As information proliferates, we’ve seen storage and cloud computing become less expensive and more commoditised. We’ve also seen AI, machine learning and data mining science become increasingly popular and widely applicable for business gain across industries.
As a result, advanced big data analytics methods such as Predictive Modeling have become more accessible for more firms. That’s good news in particular for financial services and insurance firms, plus finance departments across organisations, which can all benefit.
Predictive modeling is an increasingly popular data mining statistical technique that helps predict future behaviour by analysing historical and real-time data, then generating a model to help predict future outcomes.
In terms of helping to prevent crime and financial losses, predictive modeling can be a useful method for combatting digital fraud.
The costs of fraud are significant
Digital fraud is a real and growing problem. McKinsey uses a conservative estimate which still amounts to losses to banks alone of more than $31 billion globally in 2018. Vulnerabilities can arise from weaknesses in various digital and mobile payment platforms, while new financial solutions can sometimes harness innovative models which nevertheless provide a window of opportunity for fraudulent actors to capitalise on before “holes” are plugged. Social engineering techniques – via email or phone – and identify theft or the use of “synthetic” identifies are among the other types of many and varied digital fraud methods.
Lessening the impact of fraud through analytics and AI
But while the threat has increased, there is a positive to take away: the same technological advances that enable fraud are the same as those that can help detect and prevent it.
A 2019 report into AI and fraud published by commerce publisher, PYMNTS.com, interviewed 200 finance executives from banks and credit unions. It found that:
- 80% of fraud specialists using AI-based platforms believe the technology helps reduce payments fraud.
- 63.6% of financial institutions using AI believe it’s capable of preventing fraud before it happens, making it the most commonly cited tool for this purpose.
- The majority of fraud specialists (80%) have seen AI-based platforms reduce false positives, payments fraud, and prevent fraud attempts.
Clearly AI, analytics, and fraud specialists agree that fraud prevention methods involving data analytics and machine learning can be effective at reducing the problem.
Big data analytics has great potential in combating fraud as it can efficiently process huge volumes of data, while putting machine learning algorithms to work. By doing so, organisations can observe anomalies that occur against normal category behaviours, potentially detecting and preventing fraud on a real-time basis.
From risk assessment to managing target variables
Organisations in all sectors – and certainly in finance and insurance – now have access to more customer and transaction data that ever before and many, are beginning to integrate analytics-based decision making into company culture. Analytics and other complementary technologies can help segment customers and products by risk, which helps to ensure the focus on fraud remains in the right areas. For example, rules-based analytics can help identify low risk categories and lessen stringency around them. This in turn protects the ease and efficiency of the customer experience, wherever possible – which is a big plus for organisations seeking to balance fraud detection, security, and customer loyalty.
The key to using analytics to detect fraud ultimately comes down to the quality of your predictive fraud models. These need careful consideration, especially around the development of the target variable along with the development of the independent variables or potential predictor variables. For fraud, target variables may include whether the individual responded to an offer, made a purchase in the last X days, or made an insurance claim in the last 24 months, as examples. But fraud can be complex, so organisations need to be extremely considered – and pre-emptive rather than reactive – in identifying the behaviours which can constitute fraud, or point to its likelihood, for the analytics to be of real value. Generally speaking, “out of pattern” behaviour drives fraudulent activity – this could result from number of transactions, amounts of transactions, geographies of activity, and so on.
Ultimately, manual processes and siloed data across departments are the enemy of predictive modeling to curb fraud. A coordinated approach, an expert partner, and leaders who understand the power of advanced analytics to detect fraud are the key ingredients for success.
Antares has developed a new guide to help get started and manage big data analytics projects – such as those to prevent fraud. To download a copy, please click below.