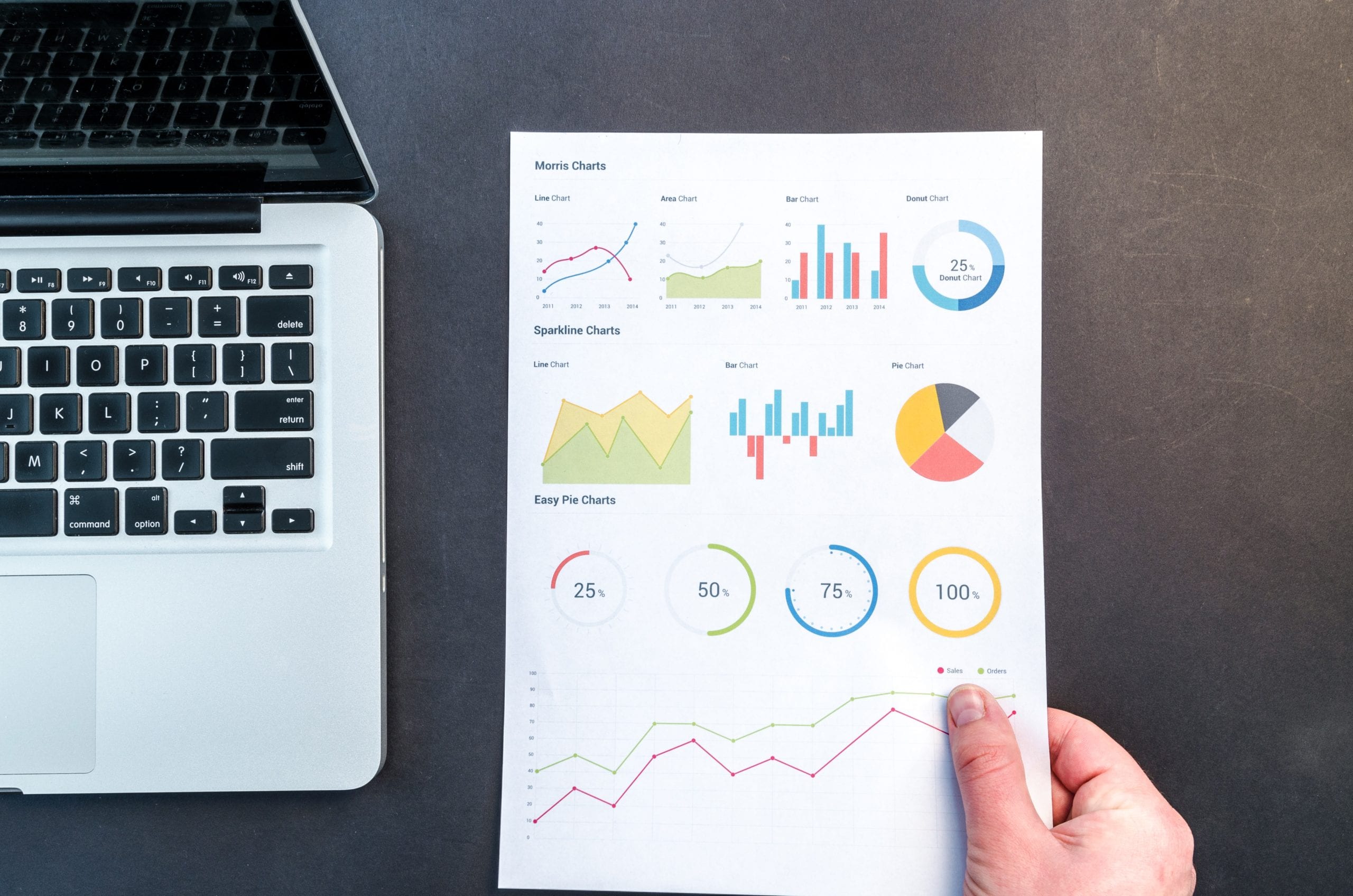
Organisation tips
What’s Stopping You from Becoming Data-Driven? Pt. 2
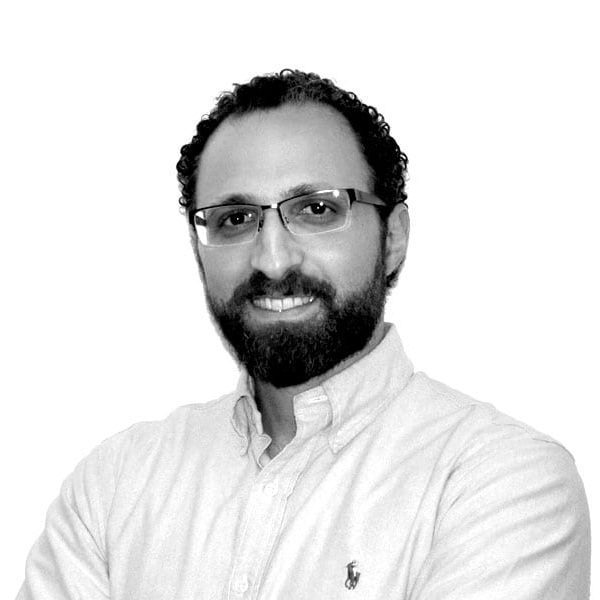
Wassim Chammaa
A seasoned Business Intelligence and Data Warehouse professional with over 15yrs of experience delivering end to end solution in Data Architecture, Analytics Statistical Analysis, and Data Mining and from Reports to Interactive Analysis or Executive dashboards.
August 22nd, 2018
In our last blog post, we shared how to address five common issues that organisations struggle with when implementing analytics solutions, including knowledge gaps and a lack of strategy. This time, we’ll focus on the top issues that can impact your potential return on investment from an advanced analytics solution. How many do you recognise from within your own business?
Issue #1: Analytics happens in siloes
The organisational structure within which an analytics capability exists can be a gamechanger in terms of successfully realising business value.
Many companies manage analytics within a reporting line below a chief data officer, who is disconnected from the day-to-day operations from the business. This approach can create risks and challenges, given the potential for organisational silos and poor coordination between data experts and business teams.
McKinsey reports that the most successful analytics investments occur in companies where the analytics and business teams work together closely in a hybrid organisational model.
Bringing together data experts and business representatives to work together as part of an agile team connects the analytics function to those accountable for achieving business value.
Issue #2: Time and effort is squandered on data management
When embarking on an analytics journey, organisations often feel pressured to do extensive data cleansing, organisation and management exercises for complete datasets first. This can be costly, time-consuming and unnecessary if not tightly focused on a specific set of use cases.
It’s true that data management is an important undertaking. But these efforts should be carefully and exclusively focused on data so they can enable use cases that are most likely to realise business value. This is precisely how we helped building supplier, GWA Group to achieve better outcomes from its data, by updating its enterprise data warehouse.
Issue #3: Striving for complete integration at the expense of other business goals
Having the right data management foundations is fundamental to successfully implementing analytics capabilities. However, this does not mean you need a single integrated data source for all company information.
Many organisations mistakenly assume that all legacy systems must be integrated into a modern platform, or that data must be housed in a single data lake before analytics solutions can be effective. This can lead to costly and time-consuming IT projects that aren’t always necessary to see ROI.
In practice, parallel data platforms will often suffice if the implementation is managed use case by use case. Talk to a data expert (Antares’ data team is always available to provide recommendations) if you’re unsure about this.
Issue #4: Business benefits of analytics can’t be measured or quantified
Analytics investments should be treated like any other – with a rigorous focus performance with quantifiable metrics to assess success or failure over the long term. Yet many companies find it challenging to define the real impact of analytics on overall business performance.
McKinsey advises that second-order metrics can be the key to success, and we tend to agree. For example, an analytics solution focused on inventory data may indicate causes of overstock. To assess the value of analytics in this case, the organisation could measure the reduction in overstock once the cause of overstock (identified by the analytics solution) was addressed.
Issue #5: Risk management approaches are not equipped for issues posed by analytics
Without applying careful attention, there is a real risk that advanced analytics encroaches on ethical, social and regulatory issues.
The ability of analytics capabilities to cluster and analyse data on sensitive variables such as gender, age and ethnicity can present:
- Significant ethical conundrums
- Legal and regulatory risks
- Broader social consequences.
Wherever analytics capabilities are in place, organisations must invest effort in establishing and maintaining effective risk management approaches that are suited to managing the unique challenges associated with analytics programs.